-- Updated on 08 January 2025 --
Simply said, video transcoding is the process of translating a video file from one format into another. This procedure is vital for media distribution since it lets videos be seen on several devices with differing capability. For example, a film that runs flawlessly on a high-end desktop could not be as good on a mobile device. Transcoding guarantees a flawless viewing by changing the video to a format the device can manage. It closes the distance between many video formats and devices like a universal translator. The reach and accessibility of video content would be much restricted without transcoding.
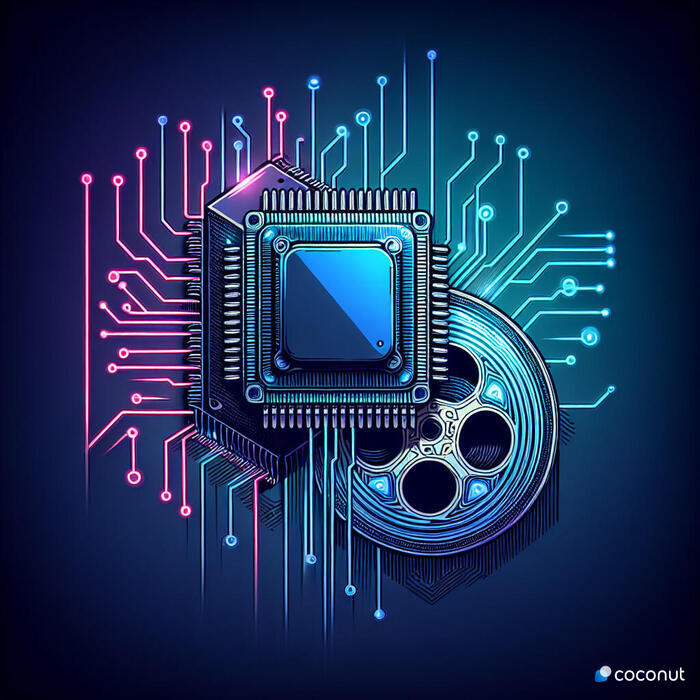
Explore the Realm of Video Transcoding
Video Codecs’ Trip From MPEG to MP4 and H.264
Video codecs have a remarkable trip of technical development in their history. Introduced in the early 1990s, MPEG-1 is the first major codec as it can compress video data without appreciable quality loss, therefore transforming video dissemination. A number of developments later resulted in the creation of MP4 and H.264 codecues. Because MP4—also known as MPEG-4 Part 14—could hold audio, video, and even subtitles in a single file, it grew rather well-known. Conversely, with its amazing compression powers, which let high-quality videos be broadcast over low-bandwidth connections, H.264, sometimes known as AVC (Advanced Video Coding), swept the planet. Modern video streaming now revolves around these codecs, which also help to enable the explosion of online video material.
Getting Through Video Transcoding’s Difficulties
Video transcoding is not without difficulties even if it is somewhat important. Keeping video quality over the compression process is one of the biggest challenges. Compression of a video results in some data loss, which naturally reduces the video quality. This is a careful balancing act; compress too little and the file size may be too great for effective streaming; compress too much and the video quality may suffer. Trans coding a video presents still another difficulty in terms of time. High-quality videos—especially in 4K or 8K resolution—can require a lot of time to convert, which would cause delays in delivery. Moreover, several devices and platforms enable various video formats, therefore adding still another level of complication to the transcoding process.
Using Artificial Intelligence in Video Transcoding
Transcoding and Artificial Intelligence: a Match Fit for Tech Heaven
Entering the field of artificial intelligence (AI), we see that it can transform video transcoding completely. AI systems can examine a video and decide intelligently on how to convert it. They can ascertain, for example, the ideal compression level to preserve video quality while keeping file size small. By determining the most effective method to transcode a movie for several devices and platforms, they also save time and money. More effective and efficient transcoding procedures result from AI learning from past transcoding activities and over time enhancing its performance. Basically, artificial intelligence provides a degree of intelligence and flexibility to video transcoding never possible in past times.
Advantages of Artificial Intelligence-Enhanced Transcoding
Examining the field of artificial intelligence-enhanced transcoding helps us to appreciate the several advantages it offers. The major benefit is the great increase in efficiency. Conventional transcoding techniques may be resource- and time-consuming. But when artificial intelligence is included into these procedures, they can be automated and simplified, therefore lowering manual intervention and accelerating turnaround times. This frees important time and lets one concentrate more on strategic chores.
Another main advantage of transcoding improved by artificial intelligence is quality optimization. Real-time video content analysis by artificial intelligence algorithms allows them to make informed recommendations regarding ideal encoding parameters. This entails choosing the correct bitrate, resolution, and frame rate to guarantee the best possible quality while so optimizing bandwidth use. Moreover, artificial intelligence may dynamically change these settings depending on device capabilities and network conditions, therefore offering the end-user an exceptional viewing experience.
Case Studies: AI in Action
Let us now review some actual case studies of how artificial intelligence has been effectively applied in transcoding systems. One such example is a top streaming service provider using artificial intelligence to improve video encoding technique. The company cut its bandwidth use by up to 20% using machine learning techniques without sacrificing video quality. Significant cost reductions and higher customer satisfaction followed from this.
One such example is a worldwide broadcast corporation that automated its video transcoding operation using artificial intelligence. The AI system was taught to identify several kinds of material and apply the suitable encoding techniques. This guaranteed constant video quality throughout all material kinds in addition to increasing the transcoding process’s efficiency.
MP4 & H.264 Transcoding Strategies
MP4 and H.264 Optimization: Foundations
Regarding MP4 and H.264 transcoding optimization, we have a few fundamental approaches at hand. Among these is selecting appropriate codec settings. Using the High Profile in H.264, for example, can yield improved quality at less bitrates over the Baseline or Main Profile. Likewise, changing the GOP (Group of Pictures) scale might have a big effect on compression efficiency and video quality.
Variable bitrate (VBR) encoding is still another fundamental method. VBR alters the bitrate depending on the complexity of the video material, unlike constant bitrate (CBR) encoding, which uses the same bitrate all through the video. Better video quality and more effective bandwidth use follow from from this.
Modern Methods of Optimization
Turning now to more advanced techniques, bitrate control and frame rate adjustment are two ways that can maximize MP4 and H.264 converting. Bitrate control is dynamic bitrate adjustment dependent on device capability and network conditions. This guarantees a flawless playback on any device and helps to avoid buffering.
Conversely, frame rate modification is lowering the frame rate for less complicated sequences or raising it for high-action scenarios. Along with reducing bandwidth, this can raise video quality. Still, it’s crucial to balance to prevent producing an odd viewing experience.
Tools for AI-driven Optimization
Additionally available are artificial intelligence solutions made especially for MP4 and H.264 transcoding optimization. These devices evaluate video footage using machine learning techniques and find the best encoding settings. Furthermore dynamically adjusting these values in real-time depending on device capabilities and network conditions is possible. Using these AI technologies would help us to attain better transcoding processes efficiency and video quality.
AI Implementation in Transcoding Systems
Connectivity with Current Systems
Integrating artificial intelligence into our present transcoding systems requires careful thought in a number of areas. Our current systems must first be made sure to be compatible with the AI technologies we intend to employ. This could call for new technology adoption or hardware or software upgrades.
We must then schedule for the integration procedure. This covers outlining the roles and duties of various team members, creating an integration schedule, and becoming ready for possible difficulties. Careful planning and execution will help us to effectively include artificial intelligence into our transcoding processes and gain from higher efficiency and quality.
AI Training for Transcoding Tasks
A crucial phase of the implementation process is teaching artificial intelligence models for transcoding optimization. This entails letting the artificial intelligence system learn from a lot of video footage and feeding it such data. Using this learning, the AI system generates intelligent decisions concerning encoding parameters including bitrate, resolution, and frame rate.
The training process calls for large computational resources and can take time. Still, the investment is well worth it since a well-trained artificial intelligence model may greatly raise our transcoding process quality and efficiency.
Evaluating AI’s Effect
It’s crucial to evaluate AI’s effects once we have included it into our transcoding processes. This entails monitoring important performance metrics including bandwidth utilization, video quality, and encoding speed. Through tracking these KPIs, we can evaluate our AI deployment’s success and make required changes to maximize our transcoding procedures even further.
AI in Video Transcoding: Future
Patterns and Anticipated Results
Looking forward, we think artificial intelligence will become ever more crucial in video transcoding. The demand for effective and high-quality transcoding solutions will only grow as video material keeps volume and complexity increasing. With its capacity to automate and maximize transcoding procedures, artificial intelligence is ideally suited to satisfy this need.
We predict the application of deep learning methods in transcoding optimization as one trend. For difficult jobs like video encoding, deep learning—a type of machine learning—can process and learn from enormous volumes of data. Deep learning will help us to raise the quality and efficiency of our transcoding processes even further.
Rising artificial intelligence-driven video analytics is another forecast. Real-time video footage analysis using artificial intelligence helps to reveal important insights about viewer preferences and behavior. These revelations create fresh chances for content producers and advertisers since they can help to customize the viewing experience and increase audience interaction.
We eagerly await witnessing how artificial intelligence will transform video transcoding as we keep discovering and using its potential. The opportunities excite us, and we are dedicated to remain leading edge technologically.
Deeper into the digital age, we find ourselves in wonder at the possibilities artificial intelligence (AI) presents to transform many fields, including video transcoding. With the ability to revolutionize our processing, storage, and distribution of video content, artificial intelligence in video transcoding looks to be quite bright. Learning and adaptability of artificial intelligence could result in more effective transcoding algorithms, therefore saving computing time and resources needed to convert videos. Given latency is a major consideration in live streaming, this could be especially helpful. Furthermore, artificial intelligence could provide more sophisticated capabilities including automatically changing quality based on viewer preferences or network conditions. Furthermore possible is the use of artificial intelligence to maximize the trade-off between file size and video quality, therefore improving viewer experiences and saving money for content producers.
New Technologies for AI Transcoding
Several future technologies could improve artificial intelligence transcoding even more. Machine learning techniques, for example, might be applied to forecast, depending on the content of a video and the viewing environment, the best transcoding parameters. Higher quality videos and better bandwidth consumption could follow from this. More complex transcoding algorithms able to adapt to a wide spectrum of video content and network conditions could also be developed using deep learning methods. Furthermore, the development of quantum computing could possibly transform artificial intelligence transcoding by offering hitherto unheard-of computational capability. This could allow even in resource-limited settings real-time transcoding of high-quality videos.
AI Transcoding: Difficulties and Considerations
Although the possibilities of artificial intelligence transcoding are fascinating, we also have to take care of several issues and considerations. The difficulty of creating and training artificial intelligence models for video transcoding is one of the primary obstacles. This calls both extensive training data and a thorough awareness of video coding standards. Furthermore, the application of artificial intelligence in video transcoding generates some moral questions. For example, how can we guarantee the transparency and fairness of the AI systems? How might viewers’ privacy be safeguarded? As we develop the field of artificial intelligence transcoding, we have to give these serious issues great thought.
Additional Readings and Internet Resources
- AI Video Generator Market Size & Trends
- AI Video Analytics Market Size
- 50 Video Statistics You Can’t Ignore In 2024