-- Updated on 30 December 2024 --
Video content has grown to be a major player in the entertainment, educational, and communication spheres of the digital age. The need of video quality assessment has become much more significant with this explosion in video usage. Simply producing and distributing video material is insufficient; the quality of the material will greatly affect viewer involvement, contentment, and finally the success of the content itself. This is where instruments for evaluating video quality—like the Structural Similarity Index (SSIM)—find application. Two photos or videos can be compared using SSIM, a technique It’s a great tool that lets video streaming companies, broadcasters, and content providers make sure their material satisfies the high standards set by discriminating consumers of today. We explore the field of video quality assessment in this paper, with specific attention to SSIM, therefore offering professional opinions on its relevance, methods, and reasons for value in the sector of video content.
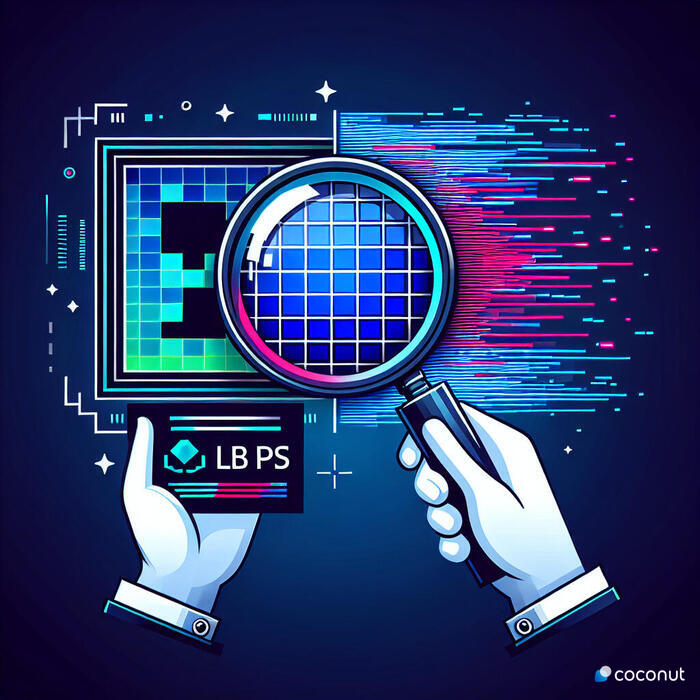
Cracking Video Quality Measurements
Anyone engaged in the production, distribution, or streaming of video content must first understand video quality criteria. They enable objective assessments and comparisons by offering a numerical value for video quality. Maintaining high standards of quality, spotting problems, and driving required improvements all depend on these measures. They also are quite important for guaranteeing a good viewing experience since bad video quality could cause viewers to be unhappy and disengaged. Knowing and applying video quality measurements is non-negotiable in an industry where success depends mostly on viewer retention and engagement.
Defining Video Excellence
One considers video quality in several dimensions. From the vantage point of the observer, it’s about the visual and aural experience: the image’s clarity, motion’s fluidity, sound and picture synchronizing. Technically, video quality encompasses several elements like bitrate, resolution, frame rate, and compression artifacts. Maintaining high visual and audio standards while controlling bandwidth and storage limitations is a difficult mix.
Examining Video Quality Measurements
The business makes use of numerous video quality measures, each having advantages and drawbacks. One often used statistic gauging the variations between the original and compressed video is Peak Signal-to– Noise Ratio (PSNR). Designed by Netflix, Video Multimethod Assessment Fusion (VMAF) is a metric combining various methods to forecast video quality. A subjective measurement based on human assessments, mean opinion score (MOS) Though none of these measurements are perfect, each offers insightful analysis of video quality. Here is where SSIM finds application.
Presenting SSIM (Structural Similarity Index)
A video quality indicator with much interest lately is the Structural Similarity Index (SSIM). Developed in 2004 by Zhou Wang, Alan Bovik, Hamid Sheikh, and Eero Simoncelli, SSIM was meant to more precisely reflect perceived visual quality, hence improving upon conventional measures like PSNR. SSIM works on the idea that the human visual system is quite suited to extract structural information from a scene, thus a measure of structural similarity can give a more accurate picture of perceived quality.
Understanding SSIM
Comparatively in terms of luminance, contrast, and structure, SSIM is a complicated method for two images or movies. The luminance comparison gauges image brightness; the contrast comparison gauges dynamic range; the structure comparison gauges pixel spatial distribution. These three comparisons then help to determine the SSIM index. SSIM is a great instrument for evaluating video quality since it can replicate the capacity of the human visual system to sense quality.
Thank You SSIM
SSIM’s application has various benefits. First of all, it offers a more realistic picture of supposed video quality than other measures. This is so considering the structural information the human visual system is natively sensitive to. Second, SSIM is a full-reference measure, so it needs comparison with the original, unprocessed image or video. This straight compares the processed video to the original, so enabling a more accurate evaluation of quality. Finally, SSIM is a reasonable choice for practical uses since it is rather simple to compute and understand.
Advantages of SSIM for Assessment of Video Quality
With numerous main advantages, the Structural Similarity Index Measure (SSIM) is a useful instrument for evaluation of video quality. SSIM first offers a more realistic depiction of apparent video quality. SSIM takes structural information, brightness, and contrast into account—more in line with the human visual system’s perception than other measures that concentrate pixel-by- pixel comparisons. This produces an honest and reasonable evaluation of video quality. Second, SSIM is quite flexible, fit for a broad spectrum of video materials and formats. From television to video game creation, this makes it an important tool for many different sectors. Finally, SSIM uses computers efficiently. For real-time video quality monitoring, it is a reasonably affordable alternative since it uses less processing capability than other video quality criteria. Many times, SSIM is chosen for video quality evaluation based on these advantages.
Constraints and Issues of Thought
Using SSIM for video quality assessment has some restrictions and considerations even if it offers benefits. One of the key constraints is SSIM might not always coincide exactly with human visual perception. Although it performs better than many other measures, there can still be differences particularly in circumstances of extreme distortion or with regard to high dynamic range content. Furthermore, not always the best option for all kinds of video material is SSIM. For evaluating the quality of videos including fast motion or sophisticated textures, for example, it might not be as successful. Moreover, even if SSIM is computationally efficient, applied to high-resolution films or vast video libraries it might still be resource-intensive. Consequently, while considering whether to apply SSIM for video quality evaluation, one must give these elements top importance.
SSIM Applied in Real-world Contextues
SSIM is extensively applied in many sectors and real-world situations for video quality evaluation. SSIM is applied in the film and television sectors post-production to guarantee color grading and visual effect quality. It enables revisions before the final work is published, so helping to discover any loss of quality that might result throughout the editing process. SSIM is used in the broadcasting sector to track the live broadcast quality. It detects any real-time decline in video quality and allows quick response to stop inadequate viewing experience. SSIM is applied in the video game sector during game creation to evaluate the animation and graphic quality. It guarantees that the game graphics satisfy the intended quality criteria, therefore improving the gaming experience. SSIM is applied in video compression to direct compression choices. It guarantees best viewers experience by helping to balance the trade-off between compression efficiency and video quality. These are only a handful of the several applications of SSIM in practical settings that show its adaptability and value in evaluation of video quality.
SSIM: Content Creation’s Approach
Regarding content development, SSIM is absolutely essential to guarantee the quality of the end result. Editors frequently have to work with the video in several ways during post-production, such color grading, visual effects addition, or scaling. Sometimes these tweaks cause video quality to drop. After every edit, SSIM can help editors evaluate the video’s quality so they may find and fix any decrease of quality. This guarantees the intended degree of quality of the last product, therefore improving the viewing experience. Moreover, SSIM allows content makers to evaluate several variations of a video, thereby guiding their decision on the one with highest quality.
SSIM in Streaming and Broadcasting
The broadcasting and streaming sectors depend critically on SSIM. Maintaining good visual quality is absolutely vital for audience enjoyment in live streaming. Real-time monitoring of the broadcast’s quality via SSIM lets one act right away should any degradation be found. This helps to avoid low-quality broadcasts causing bad viewing experience. SSIM allows one to evaluate video quality in streaming services following compression. This guarantees that, even after compression for streaming, the video keeps a high quality, therefore improving the viewing experience. Moreover, SSIM can be applied to evaluate the quality of several streams, thereby guiding service providers to select the one with the best quality.
SSIM in Compression Video
Decisions on video compression are strongly influenced by SSIM. Reducing the file size by means of video compression results in a trade-off between preserving video quality and After compression, SSIM can evaluate video quality and offer a numerical indication of the degree of degradation of the quality. This data allows one to modify the compression settings, therefore matching the demand for good video quality with the need of economy. This guarantees great quality of the compressed video, therefore improving the viewing experience. Moreover, SSIM can be applied to evaluate the performance of several compression techniques, therefore guiding the selection of the one providing the best compromise between video quality and compression efficiency.
SSIM Against Other Video Quality Measures
To grasp SSIM’s advantages and shortcomings, one commonly compares it with other video quality measures. One such statistic is the straightforward and generally used Peak Signal-to– Noise Ratio (PSNR), used in evaluation of video quality. PSNR does not take structural information, brightness, or contrast into account, though; it emphasizes pixel-by–pixel comparisons. This can cause differences between the PSNR value and the supposed video quality. Conversely, SSIM takes these elements into account and offers a more realistic picture of supposed video quality. Developed by Netflix, the Video Multimethod Assessment Fusion (VMAF) is another measure sometimes used in comparison with SSIM. To give a complete picture of video quality, VMAF aggregates numerous measures including SSIM. VMAF is more complicated and computationally demanding than SSIM even if it can offer a more thorough evaluation. Consequently, the particular needs of the video quality evaluation job will determine which of SSIM, PSNR, and VMAF is best.
SSIM Vs. PSNR
SSIM and PSNR differ in various important ways that one should take into account. A basic and easy statistic, PSNR compares the original and altered films pixel by pixel. This method does not, however, always fit human visual perception since it ignores variations in structural information, brightness, and contrast. Conversely, SSIM takes these elements into account and offers a more realistic picture of supposed video quality. Moreover, SSIM is more flexible and fit for a greater spectrum of video materials and formats. PSNR is, however, less computationally demanding than SSIM, hence it is a better fit for situations when computational capacity is restricted. Consequently, the particular needs of the video quality evaluation job determine which of SSIM and PSNR to use.
VMAF vs SSIM
Both SSIM and VMAF have advantages and drawbacks. Comprising several other measures, VMAF is a complete video quality metric. SSIM is one of them. This lets VMAF offer a more exact and thorough evaluation of video quality. VMAF is, nevertheless, also more computationally demanding and sophisticated than SSIM. Conversely, SSIM is a simpler and more effective measure that yet offers a consistent evaluation of video quality. Moreover, SSIM is more flexible and fit for a greater spectrum of video materials and formats. In other cases, though, including when handling high dynamic range material or intricate textures, VMAF could offer a more accurate evaluation. Consequently, the particular criteria of the video quality evaluation job determine the decision between SSIM and VMAF.
Developments and Prospect of Video Quality Evaluation
Video quality assessment is a field that is always changing and new technologies are routinely developing. Rising application of artificial intelligence and machine learning in video quality assessment is one of the main developments. More complex and accurate video quality measures can be developed using these methods, maybe exceeding conventional benchmarks including SSIM, PSNR, and VMAF. Moreover, the development of video quality measures capable of precisely evaluating immersive video content—including virtual reality and 360-degree videos—is under increasing importance. These kinds of materials provide special difficulties for evaluation of video quality and call for fresh methods and benchmarks. Regarding SSIM, thanks to its dependability, adaptability, and efficiency it will remain a useful instrument for evaluation of video quality. It will also have to change and adapt to the evolving scene of video content and technologies, though.
Assessment of Video Quality: Development of SSIM and Beyond
Within the field of video quality assessment, the Structural Similarity Index (SSIM) has changed everything. It provides a more precise and complete assessment than conventional techniques, therefore transforming our view and measurement of video quality. While we explore the most recent developments in video quality assessment, SSIM remains a key indicator but it is not the only one that counts. With new technologies and approaches developing and the impact of machine learning and artificial intelligence (AI) becoming evident, the terrain is changing. We will go over these advancements in this paper and consider the future course of measures such as SSIM.
Innovative Technologies for Video Quality
Video quality assessment is an ever changing field where new technologies and approaches are developing quickly. One such development is video quality prediction using deep learning algorithms. Even in intricate and dynamic situations, these algorithms can learn from vast volumes of data and generate accurate predictions regarding video quality. Cloud-based video quality assessment tools are yet another developing technology. These instruments provide scalability and adaptability that permit real-time video quality evaluation across several devices and platforms. Moreover, the introduction of 4K and 8K video formats has forced the creation of fresh measurements and techniques to fairly evaluate the quality of these high-resolution recordings. These developments are stretching the bounds of what is feasible in video quality assessment and opening the path for more exact and thorough assessments.
AI and Machine Learning’s Function
The evolution of video quality measures is being driven in great part by artificial intelligence and machine learning. These technologies can examine enormous volumes of data and find trends that humans might overlook, therefore producing more precise and complex evaluations of video quality. Common problems in compressed movies, visual distortions include blurring, blocking, and banding can be taught to machines learning techniques, for example. Conversely, artificial intelligence can be applied to create predictive models that can forecast, depending on several criteria including network circumstances and encoding parameters, the apparent quality of a video. These technologies are improving the accuracy of measurements of video quality as well as streamlining and scalability of the assessment process.
With new technologies and approaches developing and the impact of machine learning and artificial intelligence becoming evident, the terrain of video quality assessment is changing fast. Though still a crucial statistic, SSIM is no single player in the game now. Deep learning algorithms and cloud-based evaluation tools along with the development of high-resolution video formats are stretching the limits of what is feasible in video quality assessment. Still, the objective is the same: to fairly and fully assess the supposed quality of a movie in spite of these developments. SSIM is therefore still a useful technique since it provides a strong and accurate assessment of structural similarity between a reference video and a distorted video. As the area develops, though, we should anticipate fresh measurements and approaches based on the foundations set by SSIM that provide even more precise and sophisticated evaluations of video quality. We eagerly await to discover what intriguing and full of possibilities video quality assessment has in store.
Additional Studies and Online Tools
- Structural similarityindex measure (SSIM)
- An Impact of the Encoding Bitrate on the Quality of Streamed Video Presented on Screens of Different Resolutions
- Video Quality Assessment for Web Content Mirroring (PDF)