In the realm of video streaming, quality is paramount. It's the difference between a seamless viewing experience and a frustrating one. Enter Video Multi-method Assessment Fusion (VMAF), Netflix's innovative solution to video quality assessment. VMAF is a perceptual video quality assessment algorithm developed by Netflix to evaluate the quality of videos in a way that models human perception. It's a significant leap forward in the field, providing a more accurate and comprehensive measure of video quality than traditional metrics. By taking into account factors such as device type, viewing conditions, and human visual perception, VMAF offers a more holistic approach to video quality assessment. It's not just about pixel count anymore; it's about how those pixels are perceived by the human eye. This shift in perspective is revolutionizing the way we evaluate and optimize video quality, paving the way for a better viewing experience for all.

The Evolution of Video Quality Metrics
To appreciate the significance of VMAF, it's important to understand the evolution of video quality metrics. Traditional metrics such as Peak Signal-to-Noise Ratio (PSNR) and Structural Similarity Index (SSIM) have been the industry standard for years. These metrics, while useful, have their limitations. They are based on simple mathematical models and do not take into account the complexities of human visual perception. This can lead to inaccurate assessments of video quality, as they may not align with how humans perceive quality. For instance, a video with a high PSNR value may not necessarily look good to the human eye. Similarly, SSIM, while it does consider structural information in the image, does not fully capture the intricacies of human perception. These limitations underscore the need for a more sophisticated and accurate measure of video quality, a need that VMAF aims to address.
From Resolution to Perceptual Quality
The shift from simple resolution-based metrics to more complex perceptual quality assessments marks a significant evolution in the field of video quality assessment. It's a recognition of the fact that video quality is not just about the number of pixels; it's about how those pixels are perceived by the human eye. This shift is driven by advancements in technology and a deeper understanding of human visual perception. It's about moving beyond the numbers and focusing on the viewer's experience. This is where VMAF comes in. By incorporating elements of human perception into its algorithm, VMAF provides a more accurate and comprehensive measure of video quality, one that aligns more closely with how humans perceive quality.
Previous Metrics: PSNR and SSIM
Before the advent of VMAF, PSNR and SSIM were the go-to metrics for video quality assessment. PSNR is a simple, mathematical measure of the difference between the original and compressed video. It's easy to compute, but it doesn't take into account the complexities of human visual perception. SSIM, on the other hand, does consider structural information in the image, but it still falls short of fully capturing the intricacies of human perception. These metrics, while useful, have their limitations. They can provide a rough estimate of video quality, but they may not align with how humans perceive quality. This is where VMAF comes in. By incorporating elements of human perception into its algorithm, VMAF provides a more accurate and comprehensive measure of video quality.
Advantages Over Previous Metrics
The Video Multimethod Assessment Fusion (VMAF) is a significant leap forward in video quality assessment. Unlike previous metrics such as Peak Signal-to-Noise Ratio (PSNR) and Structural Similarity Index (SSIM), VMAF takes into account a variety of factors to provide a more comprehensive and accurate measure of perceived video quality. One of the key advantages of VMAF is its ability to model human visual perception. It considers factors such as contrast, detail, and motion, which are crucial in determining how humans perceive video quality. This results in a more accurate representation of the viewer's experience compared to older metrics. Additionally, VMAF is a machine learning model, which means it can continually learn and improve over time. This adaptability is a significant advantage over static metrics like PSNR and SSIM, which cannot evolve to accommodate new video technologies or viewer preferences. Furthermore, VMAF is open-source, allowing for transparency and collaboration. This openness encourages continuous improvement and innovation, which is not possible with proprietary metrics. Lastly, VMAF is versatile and can be used across a wide range of applications, from video streaming to production, making it a valuable tool in the video industry.
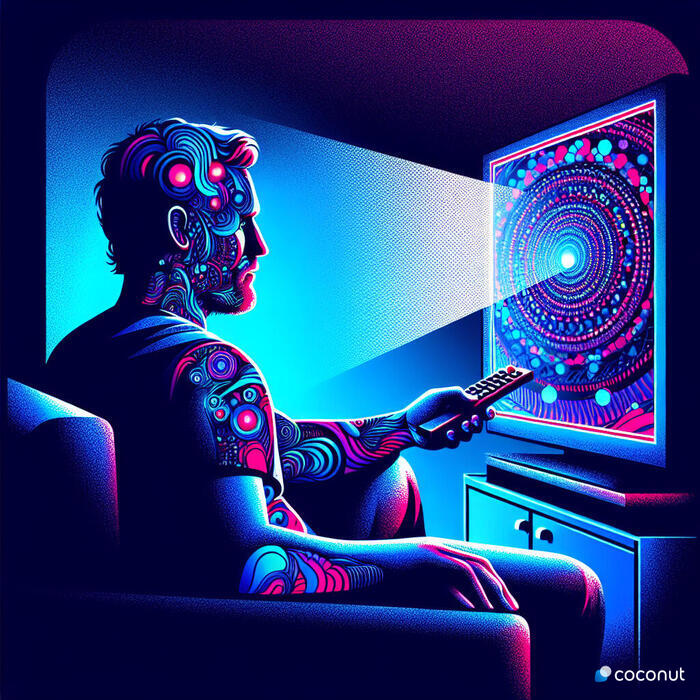
Understanding VMAF
VMAF is a perceptual video quality assessment algorithm developed by Netflix. It's designed to evaluate the quality of videos in a way that models human perception. Unlike traditional metrics, VMAF takes into account factors such as device type, viewing conditions, and human visual perception. It uses a combination of machine learning models and feature extraction techniques to assess video quality. The algorithm works by comparing a distorted video to a reference video and computing a score that represents the perceived quality of the distorted video. This score, known as the VMAF score, ranges from 0 to 100, with higher scores indicating better quality. The beauty of VMAF lies in its ability to model human perception. By incorporating elements of human perception into its algorithm, VMAF provides a more accurate and comprehensive measure of video quality, one that aligns more closely with how humans perceive quality.
Components of VMAF
VMAF is composed of several key components, each playing a crucial role in the assessment of video quality. The first component is feature extraction, where various visual features are extracted from the video. These features, which include factors such as contrast, texture, and motion, are used to model human visual perception. The extracted features are then fed into a machine learning model, which is trained to predict the perceived quality of the video. The model outputs a score, known as the VMAF score, which represents the perceived quality of the video. Another key component of VMAF is its consideration of viewing conditions and device type. By taking into account factors such as screen size, viewing distance, and device resolution, VMAF provides a more holistic assessment of video quality. This comprehensive approach sets VMAF apart from traditional metrics and makes it a powerful tool for video quality assessment.
Netflix's Role in Video Quality Assessment
Netflix has been a game-changer in the video streaming industry, and its influence extends to video quality assessment. Recognizing the limitations of existing metrics, Netflix developed VMAF to better measure video quality from the viewer's perspective. This innovative approach has shifted the industry's focus towards user-centric metrics, leading to improved video quality and viewer satisfaction. Netflix's commitment to superior video quality is evident in its rigorous testing and optimization processes. The company continuously monitors and adjusts its encoding parameters to ensure optimal video quality for every title in its vast library. This commitment to quality has set a new standard in the industry, prompting other streaming services to follow suit. Moreover, by making VMAF open-source, Netflix has fostered a collaborative environment where developers and researchers can contribute to the evolution of video quality assessment. This has led to the development of more accurate and reliable metrics, benefiting the entire industry. Netflix's role in video quality assessment is a testament to its commitment to innovation and user satisfaction, setting a benchmark for others in the industry.

Streaming Quality Challenges
Streaming video content presents unique challenges, particularly in maintaining consistent video quality. Factors such as network congestion, device capabilities, and viewer location can significantly impact the streaming experience. For instance, a viewer with a slow internet connection may experience buffering or reduced video quality. Similarly, a viewer using an outdated device may not be able to fully enjoy high-definition content. These challenges are further compounded by the sheer volume of content that Netflix streams daily. Ensuring optimal video quality for millions of viewers across different devices and network conditions is a monumental task. However, Netflix has risen to the challenge, leveraging advanced technologies like VMAF to deliver a superior viewing experience.
Netflix's Commitment to User Experience
Netflix's commitment to providing a superior user experience is at the heart of its decision to develop and implement VMAF. By focusing on perceived video quality, VMAF aligns perfectly with Netflix's user-centric approach. It allows Netflix to optimize its video content based on how viewers actually perceive quality, rather than relying on technical metrics that may not accurately reflect the viewer's experience. This ensures that viewers always receive the best possible video quality, regardless of their device or network conditions. Furthermore, by making VMAF open-source, Netflix has empowered other content providers to improve their video quality, contributing to a better overall user experience across the streaming industry.
Implementing VMAF
Implementing VMAF in video streaming and production involves several steps. First, the video content needs to be encoded using a codec that supports VMAF. Next, the encoded video is analyzed using the VMAF model to generate a quality score. This score can then be used to optimize the encoding parameters, ensuring the best possible video quality. It's important to note that VMAF is not a one-size-fits-all solution. Different content may require different encoding settings to achieve optimal quality. Therefore, it's crucial to continuously monitor and adjust the encoding parameters based on VMAF scores. Additionally, implementing VMAF requires a certain level of technical expertise. However, the benefits of improved video quality and viewer satisfaction make it a worthwhile investment.
Integration with Encoding Processes
VMAF can be seamlessly integrated into video encoding processes. It works by comparing the original video with the encoded version to assess the quality loss during encoding. This information is then used to adjust the encoding parameters, ensuring the highest possible video quality. The integration of VMAF into the encoding process allows for real-time quality assessment and optimization, making it an invaluable tool for video streaming and production.

Tools and Software Supporting VMAF
Several tools and software support VMAF, making it accessible to a wide range of users. These include FFmpeg, a free and open-source software that can encode, decode, and stream digital audio and video. FFmpeg supports VMAF, allowing users to analyze and optimize their video content. Similarly, the Moscow State University Video Quality Measurement Tool also supports VMAF. This tool provides a comprehensive suite of video quality metrics, including VMAF, making it a valuable resource for video professionals. Other tools that support VMAF include Elecard StreamEye, a professional video analysis tool, and Hybrik, a cloud-based media processing system.
Case Studies and Real-World Applications
VMAF has been successfully applied in various real-world scenarios, demonstrating its effectiveness and versatility. For instance, YouTube has used VMAF to optimize its video encoding process, resulting in significant improvements in video quality. Similarly, Twitch, a popular live streaming platform, has implemented VMAF to ensure optimal video quality for its millions of users. In the film industry, VMAF has been used to assess and optimize the quality of digital movie releases. These case studies highlight the practical applications of VMAF and its potential to revolutionize video quality assessment. As more companies adopt VMAF, we can expect to see further improvements in video quality and viewer satisfaction.
Success Stories in Streaming Services
In the realm of streaming services, the Video Multi-Method Assessment Fusion (VMAF) has been instrumental in ensuring high-quality video delivery. Netflix, a pioneer in the streaming industry, has been a notable success story. They've utilized VMAF to optimize their encoding process, resulting in a significant improvement in video quality and a reduction in bandwidth usage. Another success story is YouTube, which has leveraged VMAF to enhance their video quality while maintaining efficient bandwidth usage. The use of VMAF has allowed them to deliver high-quality videos to their users, regardless of their internet connection speed. Amazon Prime Video has also seen success with VMAF, using it to ensure the delivery of high-quality video content to their subscribers. These success stories underscore the effectiveness of VMAF in improving video quality and user experience in streaming services.
Impact on Content Creators and Broadcasters
VMAF has had a profound impact on content creators and broadcasters. It has revolutionized the way they produce and distribute content. For content creators, VMAF provides a reliable metric to assess video quality, enabling them to optimize their content for the best viewer experience. This has led to the production of higher quality content, which in turn attracts more viewers. For broadcasters, VMAF has streamlined the distribution process. It allows them to efficiently encode their content, ensuring high-quality video delivery while minimizing bandwidth usage. This not only improves viewer experience but also reduces operational costs. Thus, VMAF has become an indispensable tool for content creators and broadcasters in the digital age.

Challenges and Limitations of VMAF
Despite its numerous benefits, VMAF is not without its challenges and limitations. One of the main challenges is the complexity of its implementation. VMAF requires a deep understanding of video encoding and quality assessment, which can be daunting for those without a technical background. Additionally, VMAF is not a one-size-fits-all solution. It may not accurately reflect video quality in certain scenarios, such as low-resolution videos or videos with high motion. Furthermore, VMAF does not take into account the subjective nature of video quality. What one viewer perceives as high quality may not be the same for another viewer. This subjectivity can lead to discrepancies in VMAF scores and actual viewer experience. Lastly, VMAF is computationally intensive, which can be a limitation for real-time applications. Despite these challenges and limitations, VMAF remains a powerful tool for video quality assessment. However, it is important for users to understand these limitations and use VMAF in conjunction with other quality assessment tools and viewer feedback.
Technical Limitations
From a technical standpoint, implementing VMAF can present several challenges. Firstly, VMAF requires a significant amount of computational resources. This can be a hurdle for smaller organizations or those with limited technical infrastructure. Secondly, VMAF's algorithm is complex and requires a deep understanding of video encoding and quality assessment. This can be a barrier for those without a technical background. Lastly, VMAF is not designed to handle certain types of video content, such as low-resolution videos or videos with high motion. In these scenarios, VMAF may not accurately reflect video quality. Despite these technical limitations, VMAF remains a valuable tool for video quality assessment, provided it is used correctly and in the right context.
Future Developments and Improvements
Looking ahead, there are several potential developments and improvements to VMAF. One possibility is the integration of machine learning techniques to enhance VMAF's accuracy and efficiency. This could allow VMAF to better adapt to different types of video content and viewer preferences. Another potential development is the expansion of VMAF to handle more diverse video content, such as virtual reality or 360-degree videos. This would broaden VMAF's applicability and make it even more valuable for content creators and broadcasters. Lastly, efforts could be made to simplify VMAF's implementation, making it more accessible to those without a technical background. These potential developments and improvements could further cement VMAF's position as a leading tool for video quality assessment.
VMAF has revolutionized video quality assessment, providing a reliable and efficient tool for content creators and broadcasters. Its success stories in streaming services, such as Netflix and YouTube, underscore its effectiveness in improving video quality and user experience. Despite its challenges and limitations, VMAF has proven to be a valuable tool, provided it is used correctly and in the right context. Looking ahead, there are several potential developments and improvements to VMAF, such as the integration of machine learning techniques and the expansion to handle more diverse video content. These could further enhance VMAF's accuracy and efficiency, making it even more indispensable in the digital age. As video content continues to dominate the digital landscape, tools like VMAF will play a crucial role in ensuring high-quality video delivery and an optimal viewer experience.
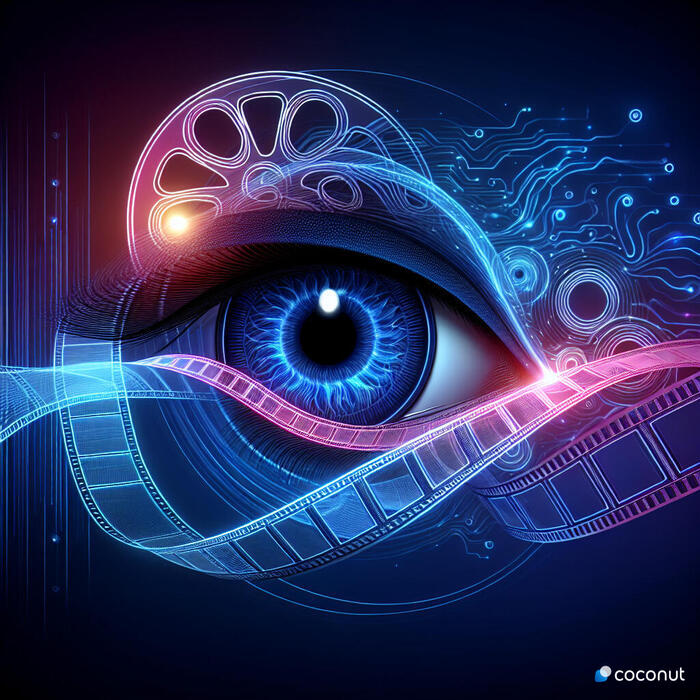